Introduction
A elementary part of statistical technique, regression evaluation is important for inspecting and measuring connections between variables. Its makes use of are quite a few and numerous, from forecasting monetary tendencies to evaluating medical outcomes. This in-depth guide explores the essence of regression evaluation, explaining its numerous sorts, purposes, and underlying ideas.
Overview
- Uncover the varied regression methods, their makes use of, and the underlying arithmetic.
- Purchase information of elementary concepts, together with the regression equation, coefficient analysis, and match metrics high quality.
- Look at the elemental presumptions of regression evaluation and their significance for reliable outcomes.
- Acknowledge the various ways in which regression evaluation could also be utilized in numerous contexts.
- Analyze the advantages and downsides of regression evaluation, contemplating its diagnostic capabilities, quantification of correlations, capability to account for confounding components, predictive energy, and limits.
What’s Regression Evaluation?
Regression evaluation is a dependable statistical methodology for ascertaining the connection between a dependent variable and a number of unbiased variables. It clarifies how modifications within the unbiased parts influence the dependent variable, making it a fundamental thought in finance, economics, and the social sciences.
Kinds of Regression
- Easy Linear Regression: As easy linear regression exhibits, a line that passes by means of the displayed knowledge factors represents the affiliation between one predictor variable and one responder variable. The target is to find out gross sales utilizing, for instance, the sum of money spent on promoting or to approximate the extent of the dependent variable numerically such that it corresponds to the extent of the unbiased variable.
- A number of Linear Regression: Multiple linear regression incorporates two or extra unbiased variables to foretell a single dependent variable, extending the capabilities of fundamental linear regression. This methodology estimates property values based mostly on dimension, location, and age and displays the cumulative affect of a number of components on the dependent variable.
- Logistic Regression: Logistic regression is used when the dependent variable is categorical or binary (e.g., true or false, sure/no). As a substitute of becoming a straight line to forecast the probability of a selected outcome, it makes use of a logistic perform (sigmoid curve). For instance, it might probably predict if a shopper will make a purchase order (sure or no).
- Polynomial Regression: Polynomial regression makes use of an nth-degree polynomial to precise the connection between the unbiased and dependent variables. By altering the predictors, it might probably now match extra intricate, nonlinear connections.
Additionally Learn: 7 Regression Techniques You Should Know!
The Regression Equation
The elemental thought is to suit a mathematical equation to noticed knowledge. In easy linear regression, the equation is:
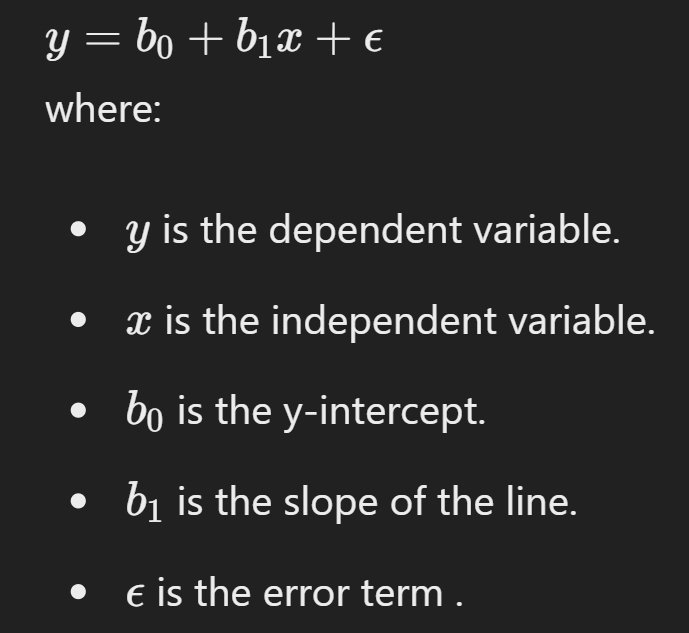
Coefficients Interpretation
The coefficients symbolize the intercept and slope. They present that the quantity of y varies when x will increase by one unit. Each unbiased variable in a number of regression has a coefficient representing its affect on the dependent variable.
Measuring Goodness of Match
- R-squared (R²): The share of the dependent variable’s volatility will be predicted based mostly on the unbiased variables. Increased R² values recommend a greater match.
- Adjusted R-squared: This method supplies a extra correct estimate in a number of regression settings by adjusting R² for the variety of predictors within the mannequin.
- P-values: Consider the coefficients’ significance. Low P-values, often lower than 0.05, point out that the affiliation is statistically important.
Assumptions in Regression
- Linearity: The connection between dependent and unbiased variables must be linear.
- Independence: Observations must be unbiased of one another.
- Homoscedasticity: The variance of errors must be constant throughout all ranges of the unbiased variables.
- Normality: One ought to usually distribute the errors.
Functions of Regression Evaluation
- Enterprise and Economics: Regression evaluation helps companies forecast gross sales, adapt costs, and seek for market indicators. It is usually used to grasp financial components like GDP and unemployment.
- Finance: They permit analysis of the threats going through a sure funding and portfolio administration by demonstrating dependency between asset costs and different variables, equivalent to rates of interest or earnings.
- Healthcare: It employs data on sufferers’ scientific and demographic knowledge to determine components related to in poor health well being. It additionally evaluates the effectiveness of therapeutic interventions and predicts affected person outcomes.
- Advertising and marketing: Regression evaluation is a technique entrepreneurs use to foretell gross sales, consider promoting campaigns, and analyze shopper habits.
- Social Sciences: Sociologists and psychologists use regression evaluation to understand the connection between variables and outcomes, equivalent to schooling and earnings patterns.
Benefits of Regression Evaluation
- Predictive Energy: This analysis’s knowledge have been analyzed by means of regression evaluation to reach on the findings associated to future outcomes. When the dependency between the variables is known, future prospects, gross sales, and different components will be calculated in particular element.
- Quantification of Relationships: It affords a exact mathematical framework for calculating the path and depth of correlations between totally different variables. This aids in comprehending how modifications to 1 variable influence these to a different.
- Management for Confounding Variables: A number of regression can embody a number of unbiased variables, which aids in figuring out one variable’s affect whereas accounting for others. That is particularly useful in difficult real-world conditions.
- Diagnostic Instruments: Regression evaluation helps with mannequin validation and enchancment by providing diagnostic instruments (equivalent to R-squared, p-values, and residual plots) to judge the mannequin’s match and the significance of predictors.
- Versatility: Regression evaluation works with numerous knowledge sorts and situations, together with steady, categorical, and binary outcomes. Furthermore, it applies to a number of professions, together with economics, engineering, and social sciences.
- Ease of Implementation: Due to fashionable statistical software program and instruments, regression evaluation is now simpler to use, even for these with out intensive statistical information. Course of simplification is achieved utilizing automated instruments in Python, R, and different platforms.
- Speculation Testing: Regression evaluation aids in testing theories in regards to the correlations between variables. It affords a construction for figuring out whether or not particular predictors considerably influence the dependent variable.
Disadvantages of Regression Evaluation
- Assumption Dependencies: A number of presumptions, together with linearity, independence, homoscedasticity, and error normalcy, underpin regression fashions. Breaking these presumptions might result in inaccurate or misleading outcomes.
- Multicollinearity: Many unbiased variables might have an effect on outcomes, making it troublesome to find out the affect of particular predictors.
- Overfitting: When educated on coaching knowledge, a mannequin overfits the coaching knowledge and performs a lot worse predicting new knowledge. This occurs when the mannequin collects noise along with the sign.
- Sensitivity to Outliers: Outliers can considerably alter the mannequin’s coefficients and outcomes in regression evaluation.
- Restricted by Linear Relationships: The belief of a linear connection between variables in easy linear regression might not maintain in all circumstances. Researchers want superior strategies like polynomial regression or machine studying fashions for extra sophisticated interactions.
- Interpretability Points: It may be difficult to find out how every predictor impacts the outcomes of a mannequin with many predictors, notably in a number of regression. This issue will increase if there are interactions between the variables.
- Pattern Dimension Necessities: Regression evaluation requires a considerable pattern dimension to yield correct estimations. Tiny pattern sizes might end in unstable estimates and insufficient generalization.
Conclusion
As a elementary device for knowledge evaluation, regression evaluation continues to offer insights and predictive energy for a variety of purposes. Nevertheless, reliability will depend on paying shut consideration to assumptions, mannequin choice, and validation, even when it affords precious instruments for forecasting and relationship comprehension.
Regularly Requested Questions
A. Regression evaluation is a statistical methodology used to grasp the connection between one dependent variable and a number of unbiased variables.
A. It tells you ways modifications within the unbiased variables are related to modifications within the dependent variable, serving to to foretell or clarify the dependent variable.
A. The principle function is to mannequin the connection between variables, permitting for predictions, insights into causal relationships, and understanding the energy of those relationships.
A. An instance is predicting an individual’s wage (dependent variable) based mostly on their years of expertise and schooling degree (unbiased variables).